Major storms are always much much worse than more typical events. In terms of power outages, they can be many orders of magnitude more impactful than typical events: sometimes cause about the same number of power outages in a day that a power utility would otherwise see over the course of a year.
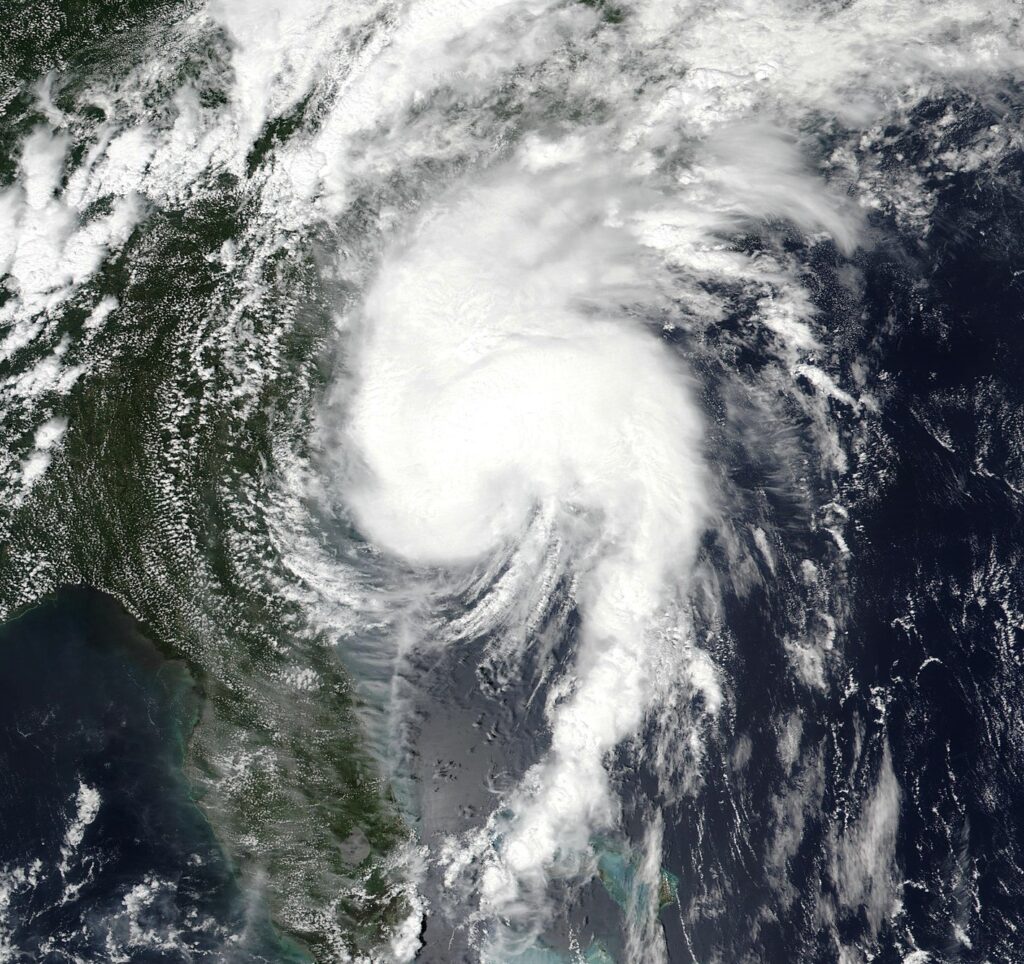
But extremes are hard to predict with machine learning for two major reasons.
- They’re rare events, and therefore you have few examples to learn from.
- Their impacts don’t fit the trends of more moderate events well and are much much larger.
But because these storms are so disruptive, they are very important to be able to predict accurately. In the journal Weather and Climate Extremes, we’ve just published a paper Improved Quantitative Prediction of Power Outages caused by Extreme Weather Events, which describes a data intensive approach of empirically predicting the impacts of storms like hurricanes on the power grid.
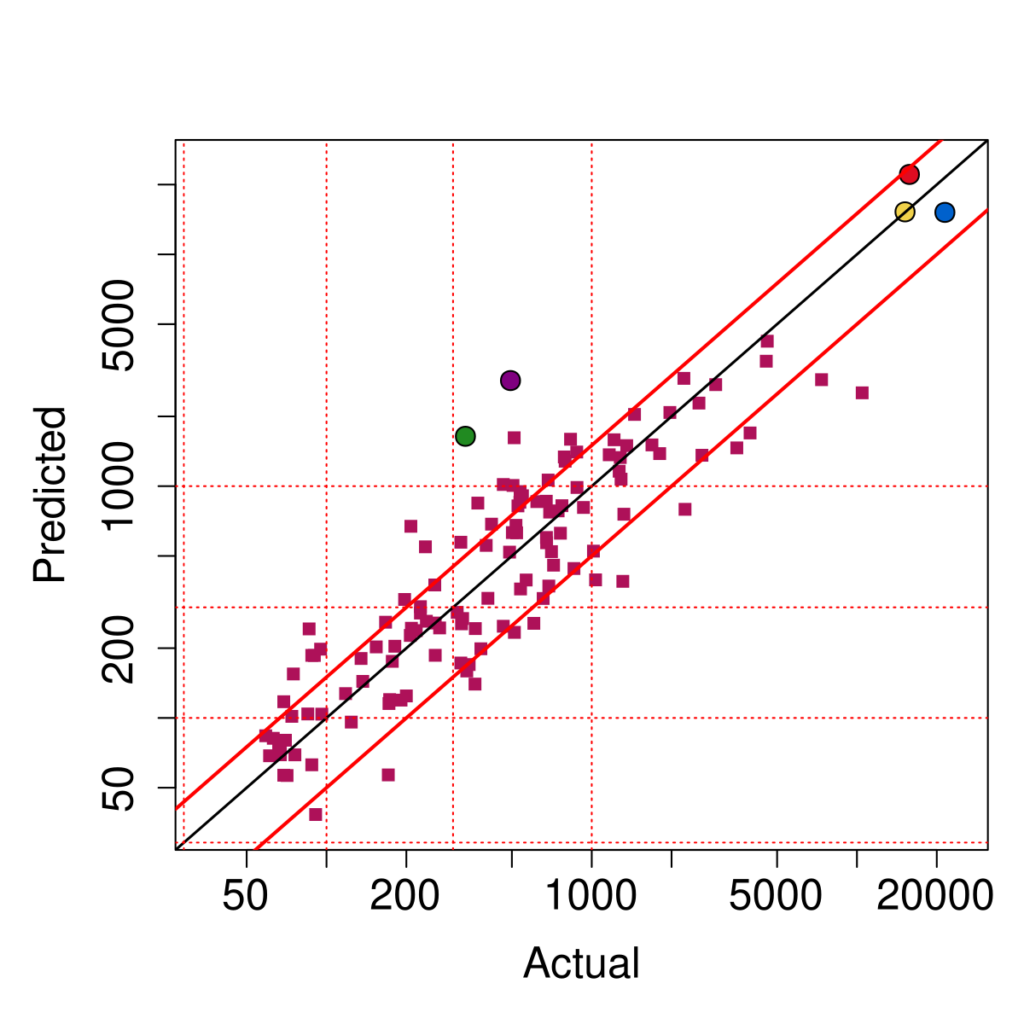
Not only do the described methods have superior accuracy, because we consider a range meteorological factors not typically considered in impact analysis we’re able to describe a diversity of factors that can contribute to the most extreme impacts of weather.
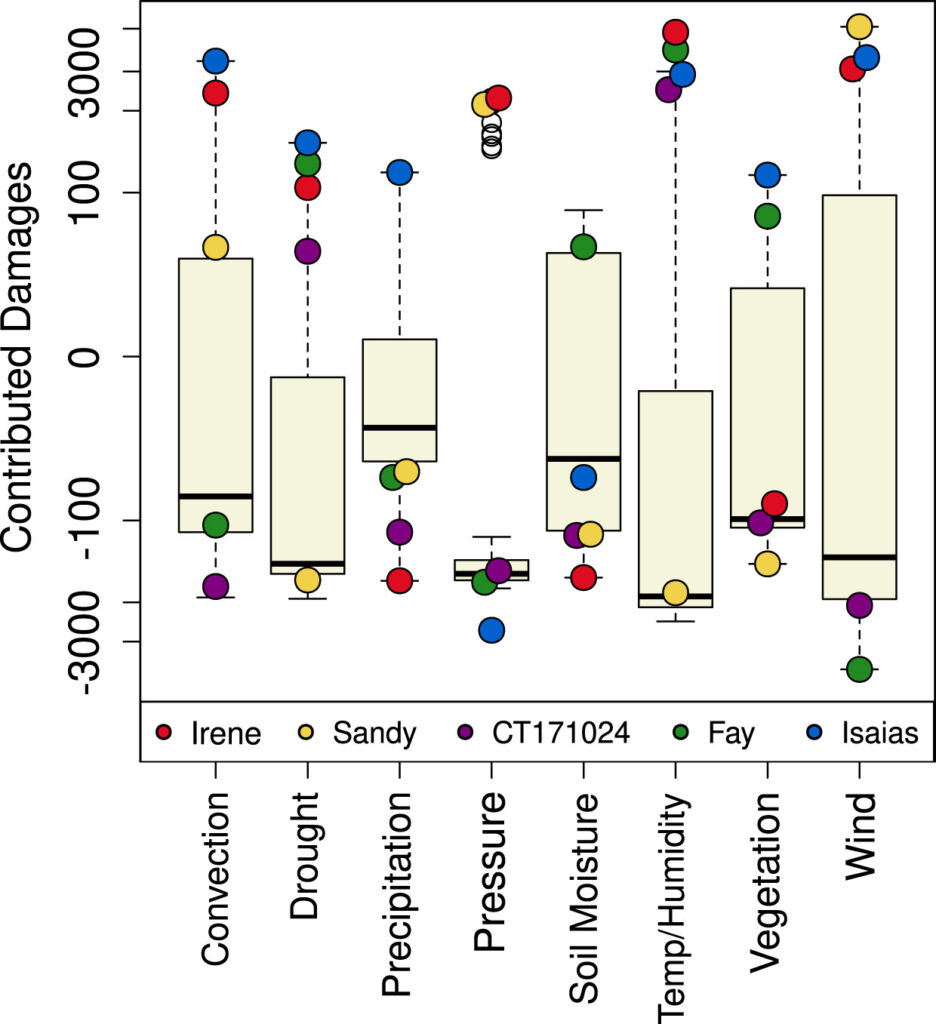